Sharper Questions
Tim Raiswell, Managing Director, Loftus Labs
Summary
Strong data analysis isn't about tools—it's about asking sharper questions. The best analysts act as a translation layer between business processes and data, refining vague problems into precise, actionable insights. This post explores that idea through a case study on labor cost analysis, showing how deeper, iterative questioning transforms surface-level observations into effective strategies.
I was recently asked to present on the topic of data analysis for the local Rotary Club. The audience comprised a mix of public and private sector professionals, both current and retired. I was forewarned that a previous guest had covered "AI and large language models," so I prepared a presentation that did not mention technology at all but instead aimed to provide practical advice on working with data.
This made me think. If asked to distinguish between poor, good, and great data analysts should your answer include any mention of the toolset they use? I hope not. My answer is: the ability of the analyst to ask sharper questions about the business problem. But what is a sharp question?
This quote (emphasis mine) is from a blog post by Roger Peng, professor of Statistics and Data Science at the University of Texas at Austin.
"The benefit of developing a sharper question is that it has a greater potential to provide discriminating information. With a vague question, the best you can hope for is a vague answer that may not lead to any useful decisions."
Avoiding Infinite Holes
Data has its own logic and exploring is fun, so unless we have a plan to guide our work, vague analysis becomes its own end. Given infinite time many data analysts will dig infinite holes. Strong analysis act as a bridge between business logic and raw data. Its success depends on sharper questions that translate vague business problems into actionable solutions.
Figure 1: Tools For Sharpening Questions
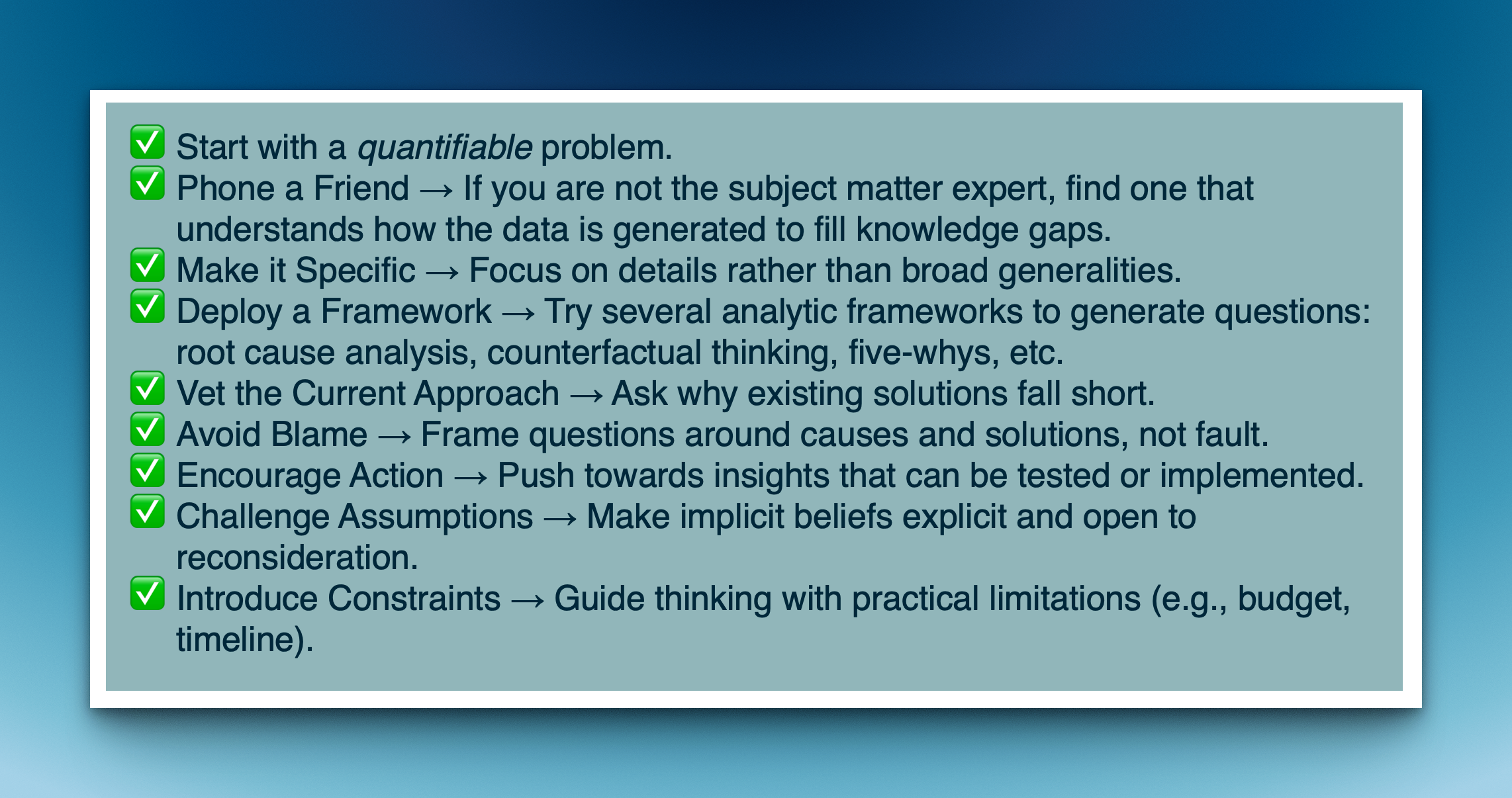
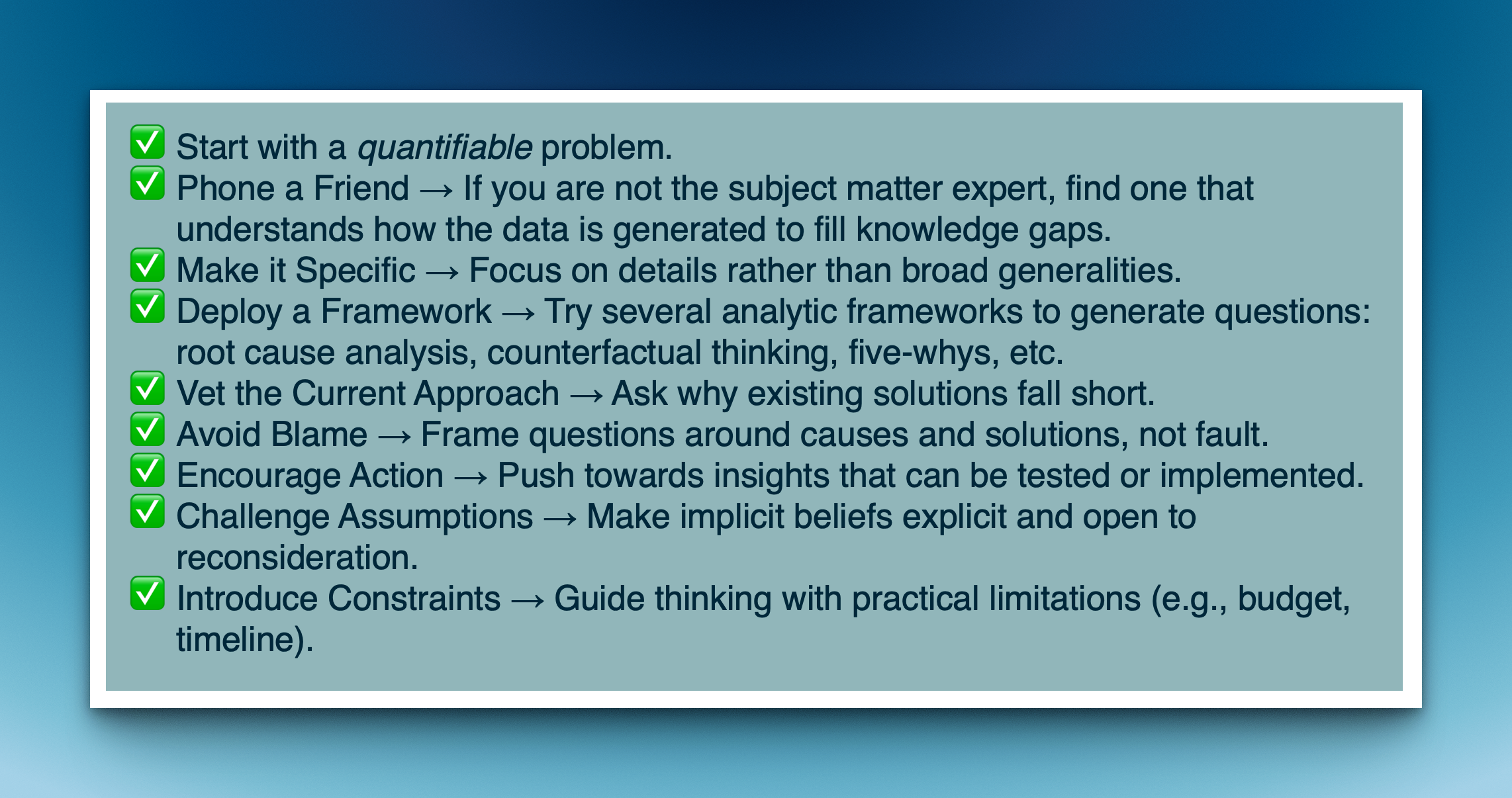
Figure 1 contains some tools for sharpening a set of analytic questions. Some of these are borrowed from Hal Gregerson's Questions Are the Answer: A Breakthrough Approach to Your Most Vexing Problems at Work and in Life (New York: Harper Business, 2018). Let's apply several of them to a labor cost analysis.
A Short Case Study in Labor Cost Analysis
Our business problem is that labor cost is too high but as a specialty crop grower you cannot mandate "Let's cut labor cost!" as it would likely result it several poor strategies that might harm critical work. Let's assume as an analyst you have spent some time with a subject matter expert and have found one controllable aspect of labor cost to be the average amount of weekly overtime incurred during the growing months of May and June. Cash spent on overtime in those months is now our target variable - one we would like to reduce.
Let's start with what seems to be a reasonable question:
Analyst (Question 1): What jobs most frequently trigger overtime? Pruning, weeding, and irrigation. Let's target those jobs first.
Subject Matter Expert (SME): Sure—but those are just common jobs in May and June, why target those?
Analyst: Because I filtered the data to show only overtime hours and these are the jobs the overtime employees are doing.
SME: Right. Those are jobs that need to be done, and if you look at those weeks, we have employees with the lowest weekly hours performing them to avoid as much overtime as possible.
Analyst (Question 2): Why is the workforce this size? Overtime is a function of constrained capacity. If you had more employees, you could avoid unnecessary overtime.
SME: Workforce size is a product of workforce planning at the beginning of the growing year. It factors in employee supply, expertise, acreage farmed, crop farmed, and existing base salary weighed against the total-cost-to-farm. I'd like to figure out if in any given week we might reduce overtime hours given a fixed workforce size.
Analyst (Question 3): How do you currently try to reduce overtime? You mentioned allocating work to employees with lower weeklyhours. Why is that insufficient?
SME: Good question. At some point close to mid-week when we know employees will start to push up against the overtime threshold, we sort our employee list by hours worked, and then look at a work plan for the rest of the week to figure out which employees are best suited given hours worked and their job skill sets.
Analyst (Question 4): Is some overtime always expected? Will there be times in the year when it is unavoidable?
SME: Yes. Workforce size is always a constraint and there are periods of time like harvest when we're trying to complete time-critical work.
Analyst (Question 5): If the work later in the week—the overtime triggering work—is necessary, is there any unnecessary work earlier in the week? What if we created a weekly work profile for overtime workers that displayed common early-week jobs that "predicted" overtime?
Review
The analyst starts with a blunt question. Their technical understanding of overtime is that it occurs when an hourly threshold is met, therefore, the culprit is the work that takes an employee over the hourly threshold.
Question 2 is an improvement in specificity and it introduces constraint-based thinking but the question drives at why any overtime exists and opens up a little more surface area than is immediately required for the problem. However, our analyst improves with Question 3 and addresses something that is important in all problem solving inquiry: how are you currently trying to solve the problem? This is a discriminating question. It drives at why the current solution is insufficient, it points to potential limitations in the data, and—as a result—it surfaces possible new solutions.
Question 4 is similar to Question 2. It is also strategic but a little sharper, as the analyst circles a new line of inquiry. If some overtime is inevitable, and there are times of the year when overtime is more likely, then perhaps there might be more than one solution. Question 5 provides the breakthrough. Overtime scenarios are not just created by late-week work, but unplanned—possibly unnecessary—early-week work. A confirmative Question 6 might ask: How common is it for skilled employees to run up time on unskilled labor earlier in the week?
Questions Act As A Translation Layer
What is implied but not explicit in our labor cost study is that effective analysts need to hold a vision of the business process in their minds simultaneously with the data model itself and translate between the two with questions. Operating with a deep understanding of just the business process or the data model will likely fail. In the case of the former, you can generate a dozen great ideas but come up short of evidence for which ones work. In the case of the latter—only using the data—it becomes an exercise in vague, abstract thought with no resulting action taken.
Get some time with one of our agribusiness analytics experts to learn more via our contact page.